Overview
For this project, I wanted to dive into something really important to me: individual differences. So much of research involves reducing a diverse group of people to a single average. This is usually necessary if we want our findings to be interpretable and actionable, but it comes at a cost. Every part of the individual that we ignore decreases the precision of our model and the impact of our decision.
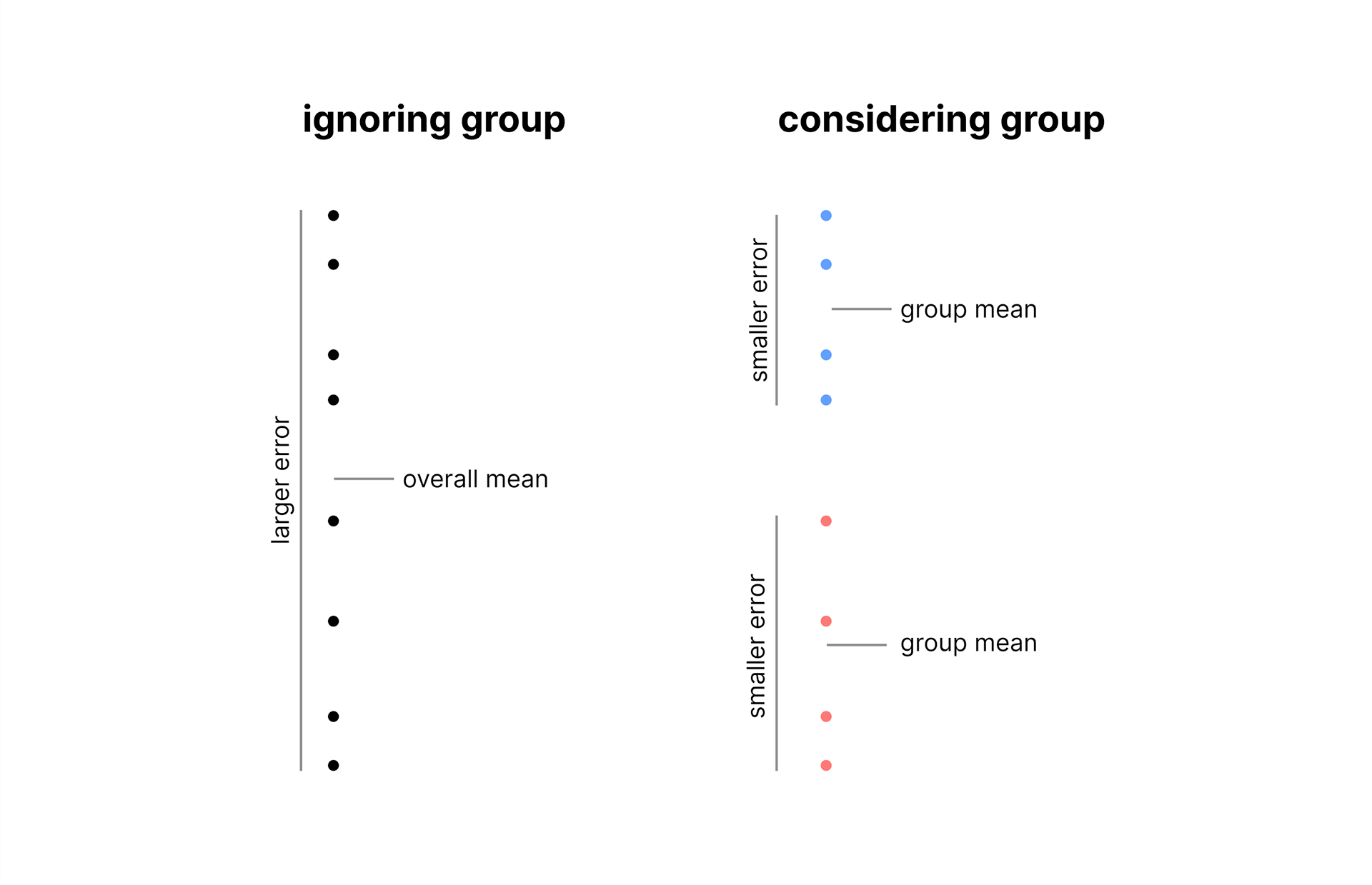
So how do we strike a balance? Consideration of group differences is a good place to start. The purpose of this analysis was to see if the structure of a psychometric scale depends on gender. Spoiler, it does.
Analysis
I analyzed the Generic Conspiracist Beliefs Scale (GCBS), a 15-item scale measuring five domains of conspiratorial beliefs. This framework was developed by Brotherton, French, & Pickering in 2013.
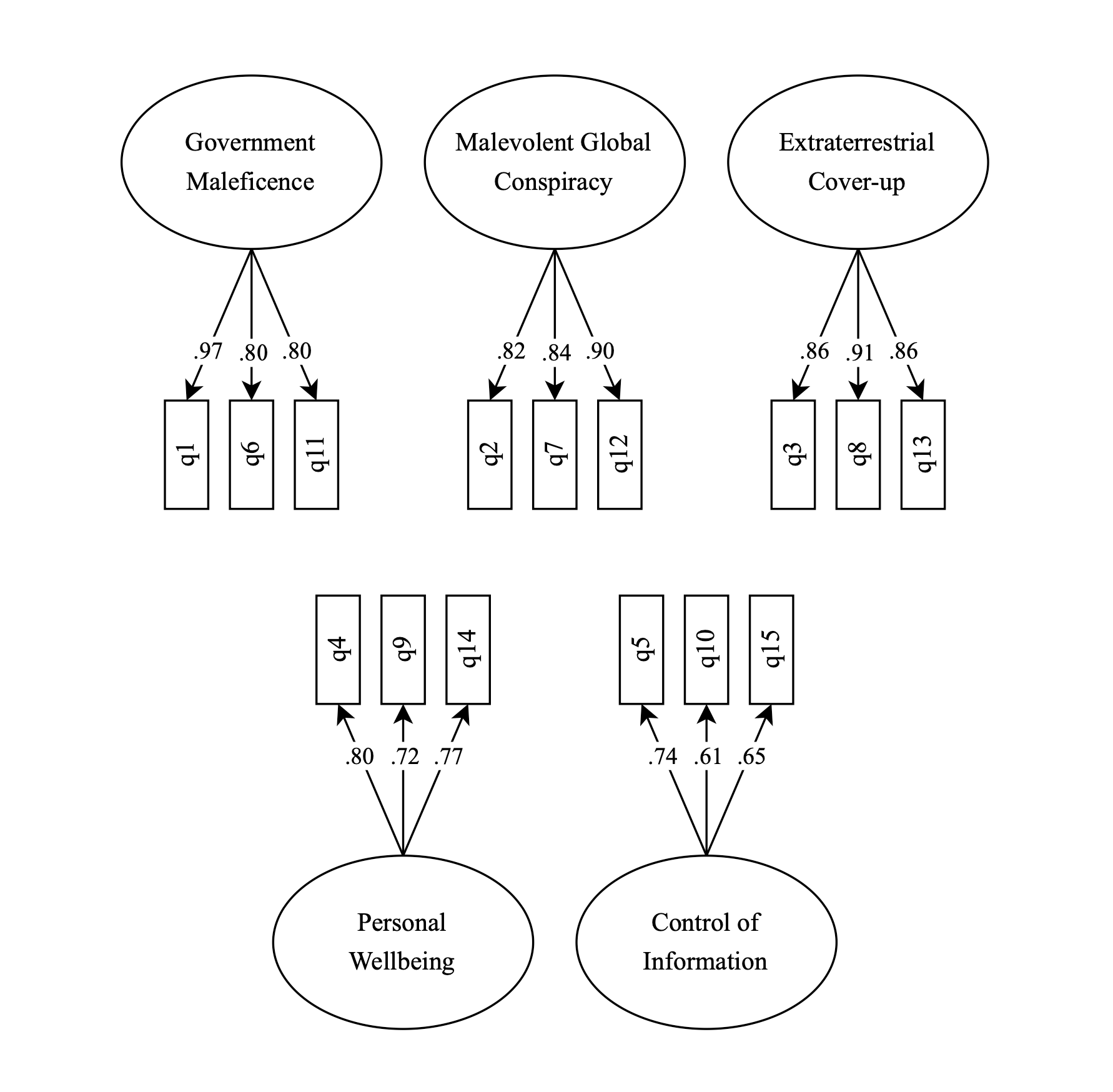
The researchers checked several types of reliability and validity after they developed this framework, but they didn’t assess the degree of "measurement invariance," or lack of difference in the structure of the framework, between groups. A scale with stronger measurement invariance is usually considered better, since that means it measures the same thing, the same way, no matter who you give it to.
Methods and Results
I used two factor analyses to identify and address gender-based differences in this scale. A factor analysis is a way of using observed variables to find latent variables. Observed variables are things that we can directly measure, like test questions and survey items. Latent variables are things that we can't directly measure, but that impact observed variables. Personality traits and intelligence are good examples of latent variables.
We can use observed variables to find latent variables. This involves seeing how the observed variables in a dataset "covary," or move together. Observed variables that strongly covary are assumed to belong to the same latent variable. A factor analysis optimizes the way observed variables are clustered to maximize the covariance within each latent variable. A "factor loading" is calculated for all of the observed variables. This loading tells us how strongly an observed variable is influenced by a latent variable.
I found a large dataset on the Open Source Psychometric Project with GCBS item responses and demographic information for each participant. I used a Multi Group Confirmatory Factor Analysis to test the original structure of the GCBS. This revealed no measurement invariance, suggesting substantial gender-based differences in the structure of conspiratorial beliefs. To find better structures that capture these differences, I conducted two Exploratory Factor Analyses, one on men and one on women.
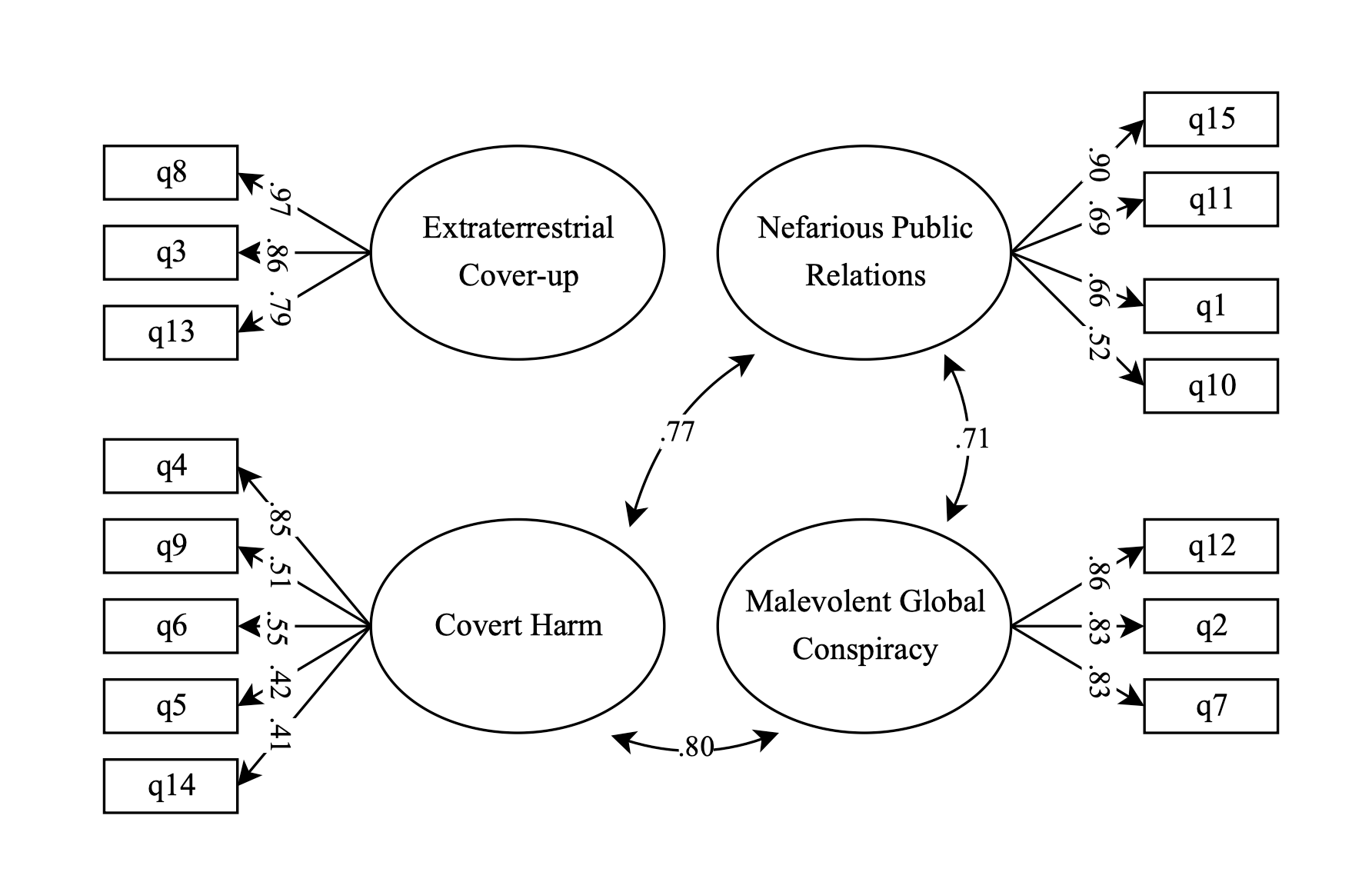
Structure of conspiratorial beliefs for men
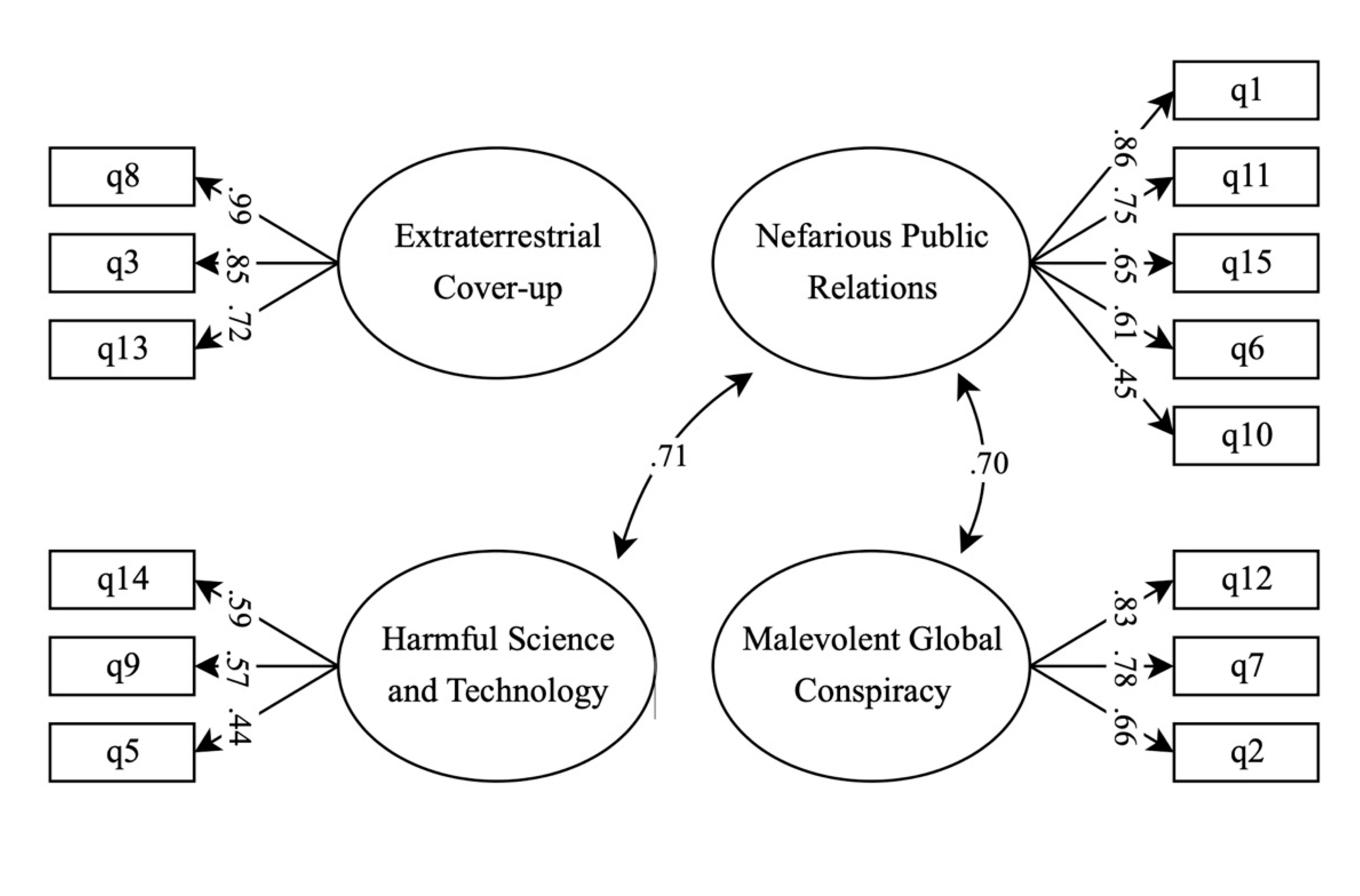
Structure of conspiratorial beliefs for women
There are two notable differences in these structures: domain size and domain theme. For men, the largest domain of conspiratorial beliefs is related to harm (Covert Harm), while for women the largest domain is related to deceit (Nefarious Public Relations).
While both genders have a domain related to harm, the scope of this harm differs. For men, the harm domain (Covert Harm) contains a variety of beliefs related to a generalized sense of harm. For women, the harm domain (Harmful Science and Technology) contains only specific beliefs related to science and technology. These structures vary because men and women differed in their evaluation of two ambiguously-worded items. For men, these items were associated with items related to harm; for women, they were associated with items related to deceit.
Discussion
There are probably lots of reasons for this difference in structure. It may be due in part to differences in threat perception. Compared to women, men score higher on measures of reactive aggression and have stronger emotional responses to ambiguous social stimuli (Im et al., 2018; Newhoff et al., 2015). This could explain why ambiguous beliefs were perceived by men as primarily harmful and by women as primarily deceitful.
More than anything, this project was an exercise in measurement refinement. The analysis took under an hour and provided important insights about how different types of people see the world. Scale development is an iterative process — an empirical framework is a valuable tool, but to get the most out of it, we have to consider and address its limitations. With just a few minutes and some really cool stats, we can find and fix shortcomings to make our research more precise and useful.